Table Of Content

There are even laws in the federal code about the use of research data. Clearly, it is never acceptable to falsify data the way this researcher did. Notice that the lurking variable, age, can also be a contributing factor. While there is a correlation between smoking and mortality, and also a correlation between smoking and age, we aren’t 100% sure that they are the cause of the mortality rate in women. The issue of measurement bias arises due to unrecognizable differences in the evaluation process. In an investigation of the effects of education on income, the factor being studied is education level (qualitative but ordinal).
Statistical experiments, following Charles S. Peirce
Double-blind study is where neither the individual nor the researcher knows who is getting which treatment or who is getting the treatment and who is getting the placebo. This is important so that there can be no bias created by either the individual or the researcher. Repetition of an experiment on more than one subject so you can make sure that the sample is large enough to distinguish true effects from random effects. It is also the ability for someone else to duplicate the results of the experiment.
Table of contents
It involves carefully designing an experiment that can test the hypothesis, and controlling for other variables that may influence the results. For example, imagine we want to study if walking daily improved blood pressure. What is important to note about the difference between confounding and lurking variables is that a confounding variable is measured in a study, while a lurking variable is not.
The Multiple Testing Problem
Experiments are always context-dependent, and a good experimental design will take into account all of the unique considerations of your study system to produce information that is both valid and relevant to your research question. Finally, you need to decide how you’ll collect data on your dependent variable outcomes. You should aim for reliable and valid measurements that minimise bias or error. How you manipulate the independent variable can affect the experiment’s external validity – that is, the extent to which the results can be generalised and applied to the broader world. Experimental research design should be used when a researcher wants to establish a cause-and-effect relationship between variables.
Step 1: Define your variables
Probably many factors, temperature and moisture, various ratios of ingredients, and presence or absence of many additives. Now, should one keep all the factors involved in the experiment at a constant level and just vary one to see what would happen? Then measure your chosen response variable at several (at least two) settings of the factor under study. If changing the factor causes the phenomenon to change, then you conclude that there is indeed a cause-and-effect relationship at work. How precisely you measure your dependent variable also affects the kinds of statistical analysis you can use on your data. Experimental design also allows researchers to generalize their findings to the larger population from which the sample was drawn.
Power and optimal study design in iPSC-based brain disease modelling Molecular Psychiatry - Nature.com
Power and optimal study design in iPSC-based brain disease modelling Molecular Psychiatry.
Posted: Wed, 16 Nov 2022 08:00:00 GMT [source]
One or more of these variables, referred to as the factors of the study, are controlled so that data may be obtained about how the factors influence another variable referred to as the response variable, or simply the response. As a case in point, consider an experiment designed to determine the effect of three different exercise programs on the cholesterol level of patients with elevated cholesterol. The two conditions were treated exactly the same except for the instructions they received. Therefore, it would appear that any difference between conditions should be attributed to the treatments themselves.
Rigorously Controlled Design:
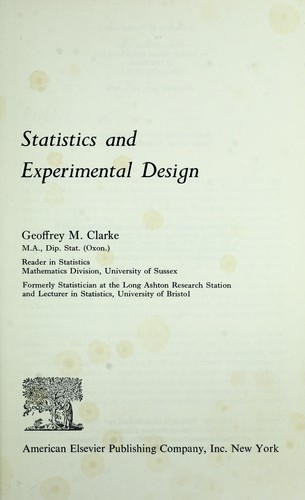
The quality of your sampling and the design of your experiment can have an effect upon the outcome and determine whether your hypothesis is rejected or not. Therefore it is important to have an appropriate and properly designed experiment. Within-subjects or repeated measures can also refer to an experimental design where an effect emerges over time, and individual responses are measured over time in order to measure this effect as it emerges. In a between-subjects design (also known as an independent measures design or classic ANOVA design), individuals receive only one of the possible levels of an experimental treatment.
The same is true for intervening variables (a variable in between the supposed cause (X) and the effect (Y)), and anteceding variables (a variable prior to the supposed cause (X) that is the true cause). When a third variable is involved and has not been controlled for, the relation is said to be a zero order relationship. In most practical applications of experimental research designs there are several causes (X1, X2, X3). This involves randomly assigning participants to different groups or treatments to ensure that any observed differences between groups are due to the treatment and not to other factors. Is one brand of fertilizer more effective at growing roses than another? In this module, you will learn important aspects of experimental design.
This experimental design method involves manipulating multiple independent variables simultaneously to investigate their combined effects on the dependent variable. In a factorial design, participants are randomly assigned to one of several groups, each of which receives a different combination of two or more independent variables. The subjects were observed for a year, and the number of seizures for each subject was recorded.
Each group receives a different level of the treatment (e.g. no phone use, low phone use, high phone use). First, you may need to decide how widely to vary your independent variable. Experimental design means creating a set of procedures to systematically test a hypothesis. A good experimental design requires a strong understanding of the system you are studying.
It is particularly useful when studying the impact of an intervention or treatment on a particular outcome. Physiological measures involve measuring participants’ physiological responses, such as heart rate, blood pressure, or brain activity, using specialized equipment. These measures may be invasive or non-invasive, and may be administered in a laboratory or clinical setting. Behavioral measures involve measuring participants’ behavior directly, such as through reaction time tasks or performance tests. These measures may be administered using specialized equipment or software.
A model of the relationship is hypothesized, and estimates of the parameter values are used to develop an estimated regression equation. Various tests are then employed to determine if the model is satisfactory. If the model is deemed satisfactory, the estimated regression equation can be used to predict the value of the dependent variable given values for the independent variables. The purpose of experimental design is to control and manipulate one or more independent variables to determine their effect on a dependent variable.
Self-report measures involve asking participants to report their thoughts, feelings, or behaviors using questionnaires, surveys, or interviews. Replication involves conducting the same experiment with different samples or under different conditions to increase the reliability and validity of the results. Blinding involves keeping participants, researchers, or both unaware of which treatment group participants are in, in order to reduce the risk of bias in the results. In this design, each participant is exposed to all of the different treatments or conditions, either in a random order or in a predetermined order. For example, if it is surmised that a new medicine reduces the effects of illness from 72 hours to 71 hours, this would not be considered statistically significant.
Experimental design allows researchers to systematically investigate causal relationships between variables, and to establish cause-and-effect relationships between the independent and dependent variables. Through experimental design, researchers can test hypotheses and make inferences about the population from which the sample was drawn. Please note that our video lesson will not focus on quasi-experiments. A quasi experimental design lacks random assignments; therefore, the independent variable can be manipulated prior to measuring the dependent variable, which may lead to confounding. For the sake of our lesson, and all future lessons, we will be using research methods where random sampling and experimental designs are used. For example, subjects can all be tested under each of the treatment conditions or a different group of subjects can be used for each treatment.
Thus the second experiment gives us 8 times as much precision for the estimate of a single item, and estimates all items simultaneously, with the same precision. What the second experiment achieves with eight would require 64 weighings if the items are weighed separately. However, note that the estimates for the items obtained in the second experiment have errors that correlate with each other.
No comments:
Post a Comment